ConcertAI’s team of senior machine learning engineers continue to develop advanced rules-based and predictive AI technologies for healthcare. See their latest research using AI for clinical research and real-world evidence.
ASCO 2020
Deep Learning Model Using NLP to Identify Metastatic Status from Unstructured Notes
This study was conducted to determine if contextual understanding of metastatatic status can be extracted automatically from physician notes to identify patients with metastatic breast cancer.
Read More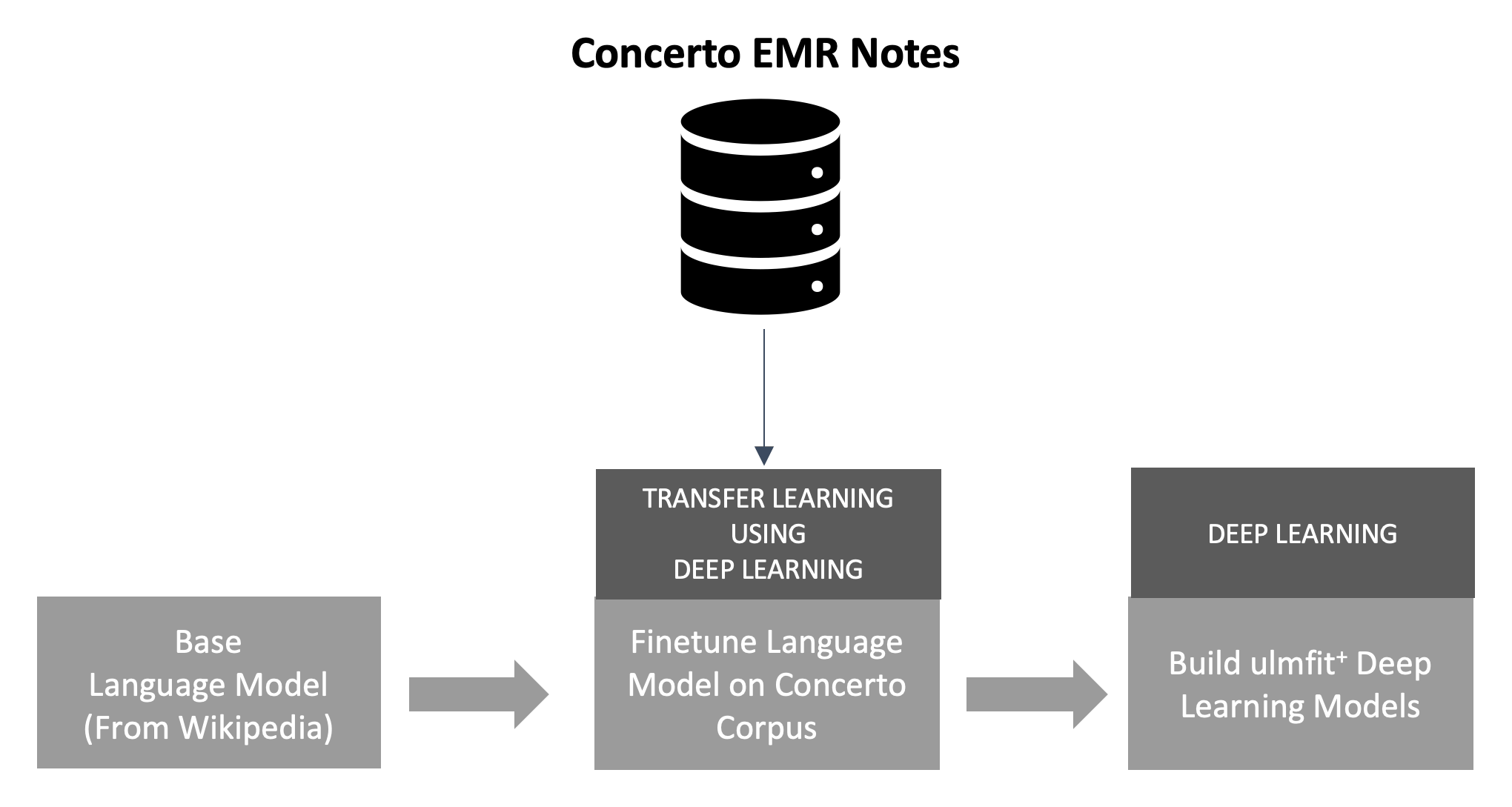
Snapshot of methodology to train deep learning models
ASCO 2020
AI Model to Predict Slow Progressors
There are ongoing efforts to understand and predict exceptional response to existing cancer therapies, but few clinical characteristics of these patients are known. We trained a machine learning model using the ConcertAI database of oncology EMR data that includes clinical data from CancerLinQ Discovery to predict slow progression, a proxy for exceptional response, in aNSCLC in the second line setting.
Read More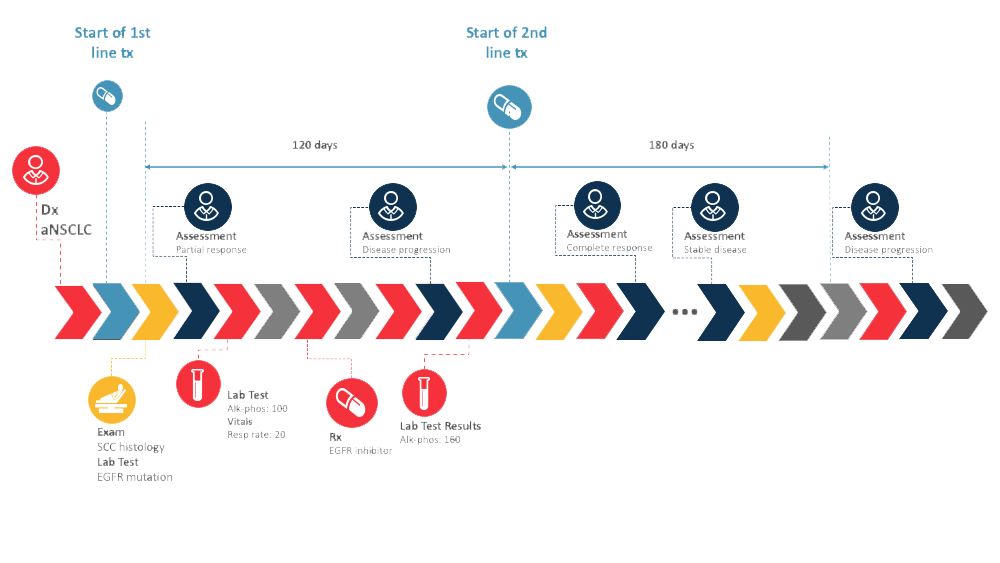
AI-based assessment of progression
ASCO 2020
Dynamic Model to Predict Metastatic Recurrence
Machine Learning models that can dynamically predict risk of metastatic breast cancer (mBC) based on cumulative historical clinical data could help guide patient care and monitoring decisions. This study looked at an ML model that sought to predict risk of recurrence at any point in the patient journey.
Read More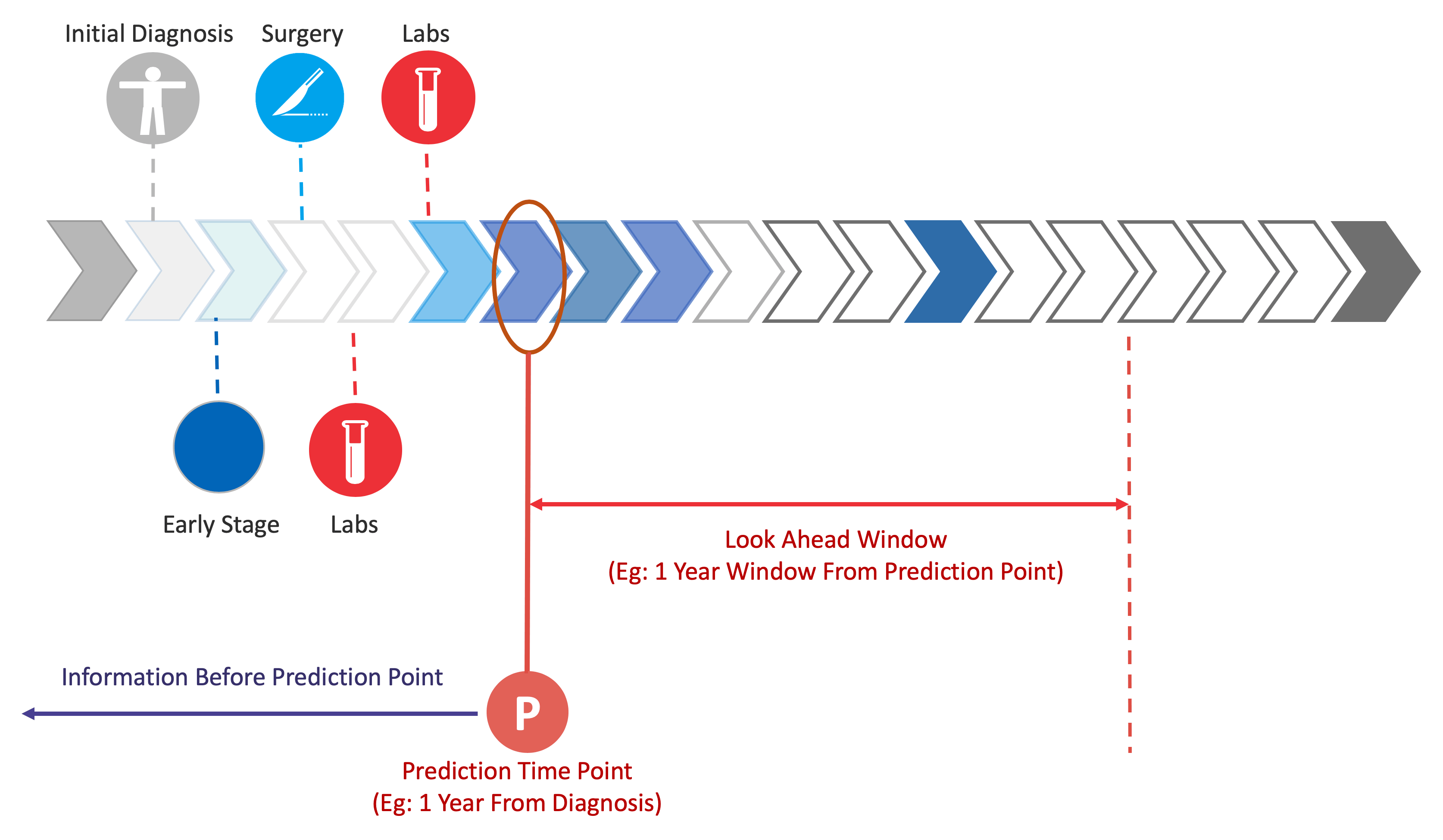
Depiction of a patient journey and features used to understand risk of recurrence
ISPOR 2020
ML Model to Impute Cancer Subtype
Ability to distinguish between subtypes of lung cancer (LC) is important for clinical outcomes and cost analysis, but this information is seldom captured in the structured electronic health record (EHR) data. The objective of this study was to develop and validate an artificial intelligence model to identify non-small cell lung cancer (NSCLC) patients from a cohort of heterogeneous LC patients using de-identified retrospective EHR data.
Read More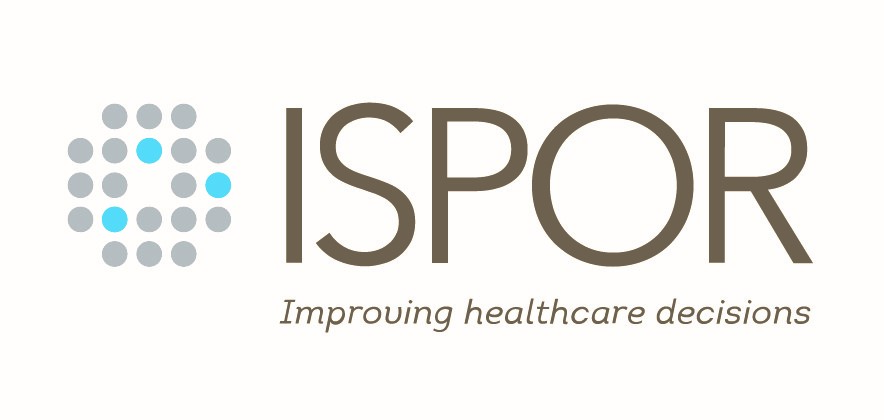
ISPOR 2020
AI Model to Predict Cardiac AEs
Many oncology treatments have been associated with cardiovascular (CV) adverse events. We created a machine learning model to predict potential CV events in PD-(L)1 patients using the CancerLinQ database.
Read More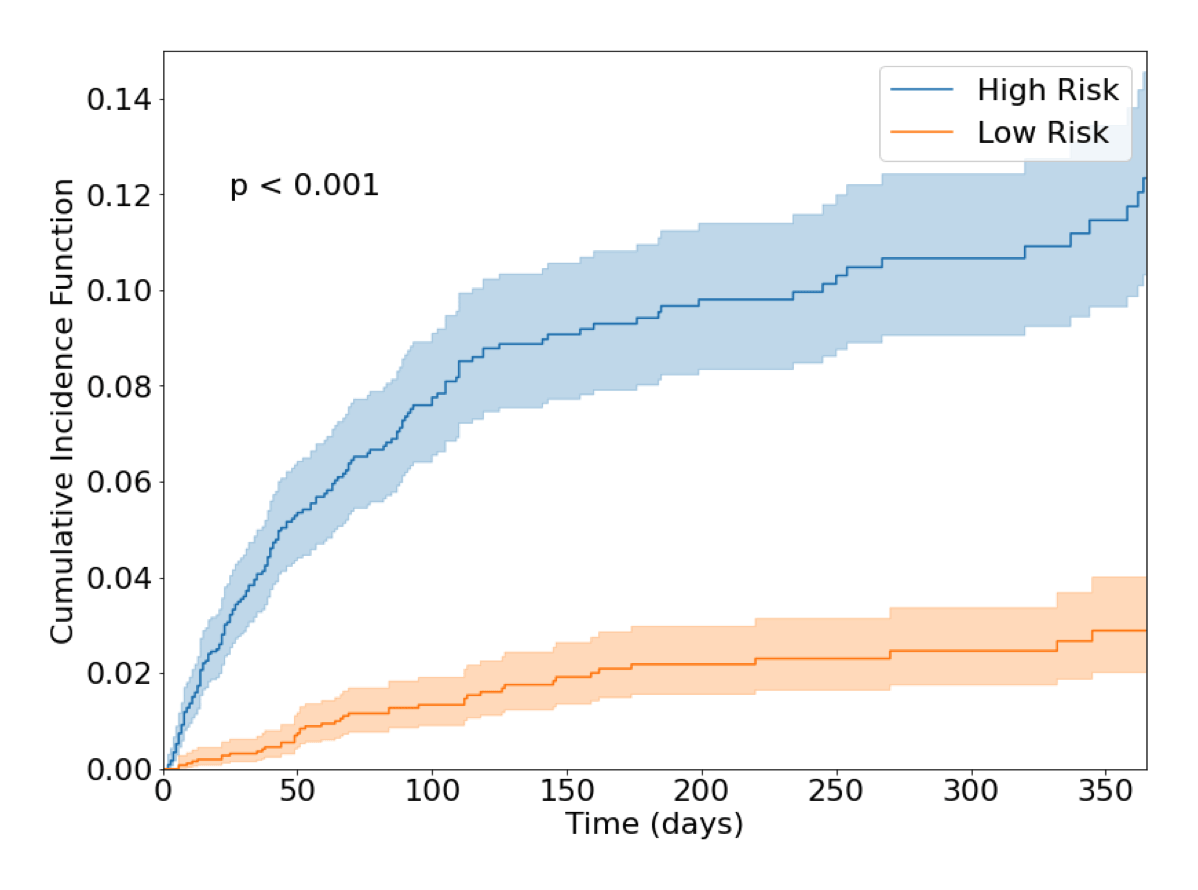
Risk Prediction Model
ASCO 2020
AI Model to Impute ECOG Scores
COG PS is a prognostic indicator of outcomes, and scores of 0-1 (good ECOG PS) are often required for clinical trial enrollment. Patients treated in non-trial settings often lack ECOG PS scores limiting the ability of Real World Data from these patients to be used in external control arms (ECAs) or to provide optimal specificity for clinical effectiveness research. We developed a series of machine learning models to impute ECOG PS at initial diagnosis, metastatic diagnosis and final evaluation.
Read More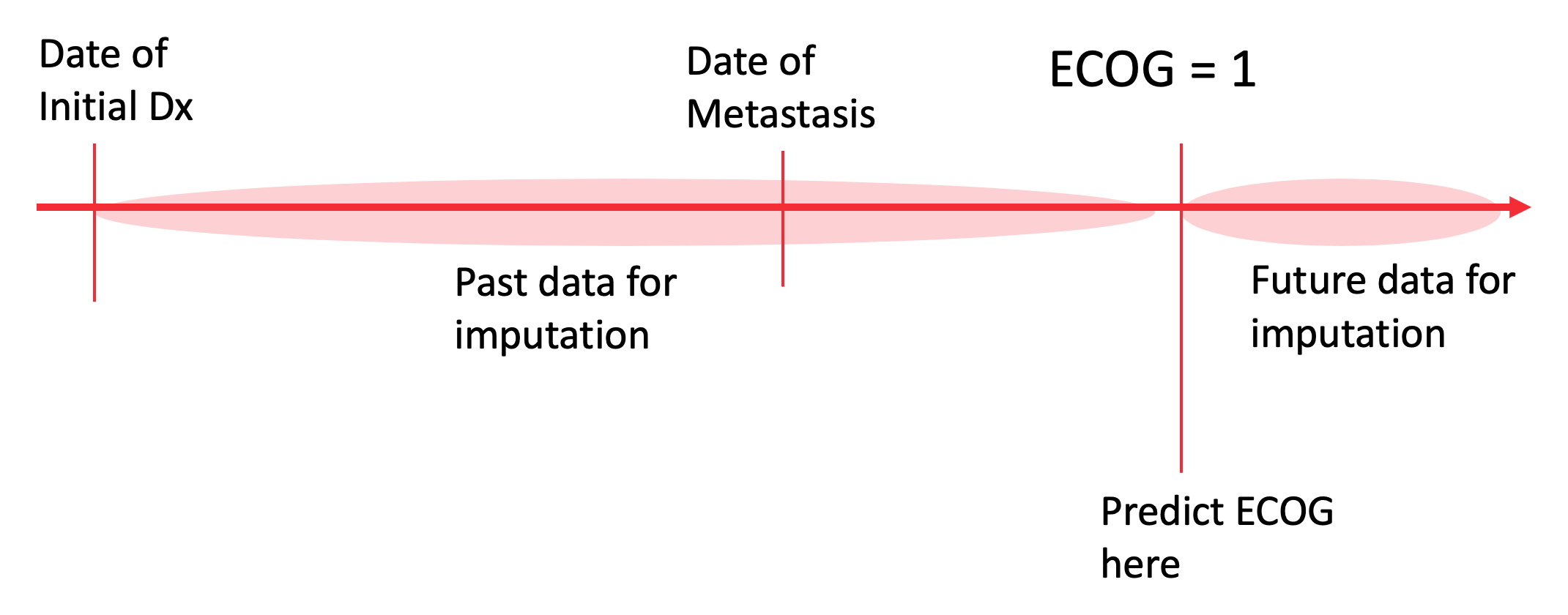
ECOG Imputation Modeling
ASCO 2019
Predicting Survival at Specific Time Intervals
Survival prediction models for lung cancer patients could help guide their care and therapy decisions. We built a machine learning model to predict probability of survival beyond 90, 180 and 360 days from any point in a lung cancer patient’s journey.
Read More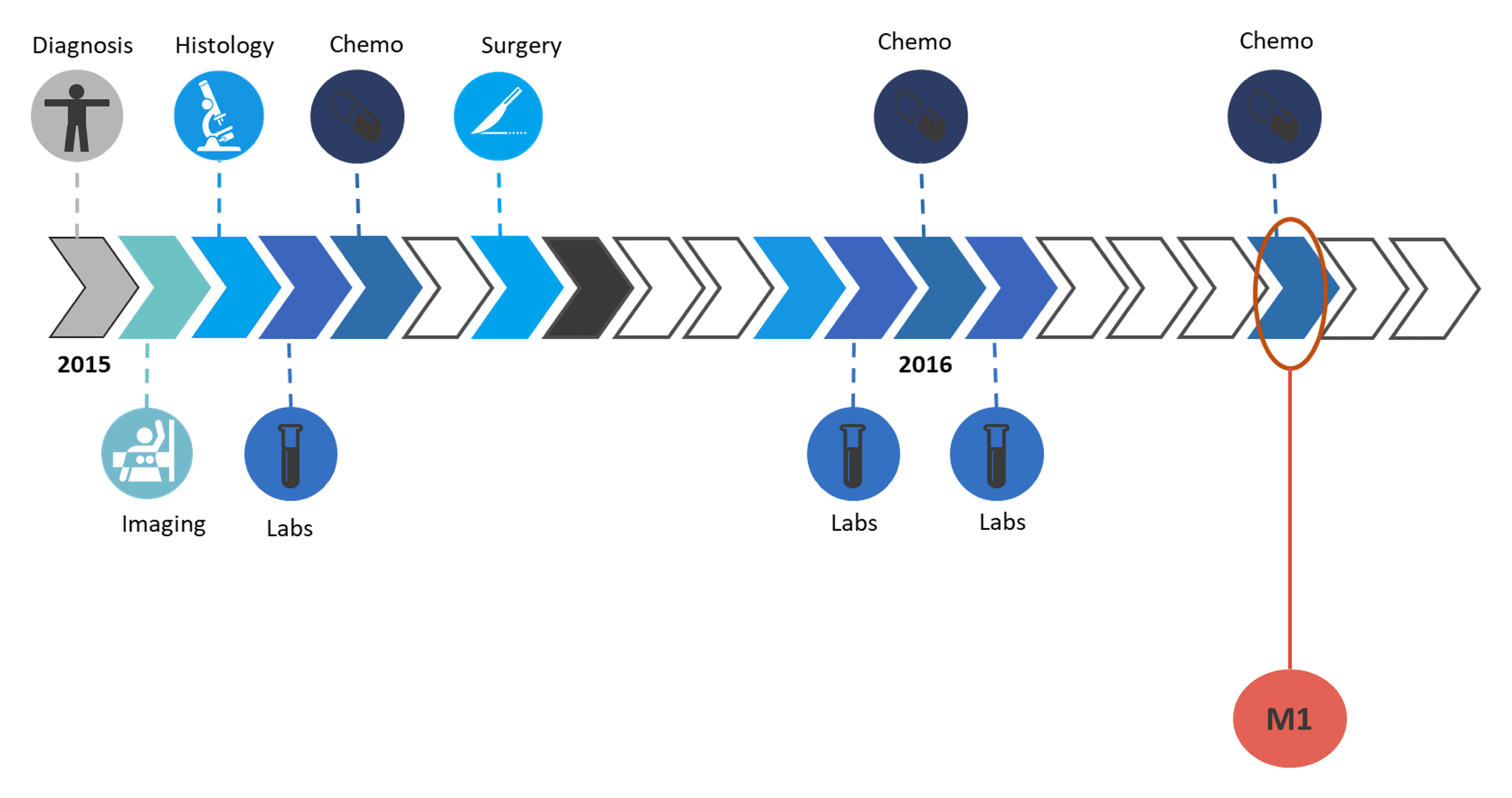
Depiction of a patients journey as captured in the EHR and the features used to build the model
ISPOR 2019
AI Model to Improve Capture of Metastatic Status from EMR
Though an important prognostic feature in cancer, stage information is often missing from patient’s EHRs and unavailable in claims data. We developed and validated an AI model that classifies metastatic status in BC patients at their last observed timepoint (proxy for present-day).
Read More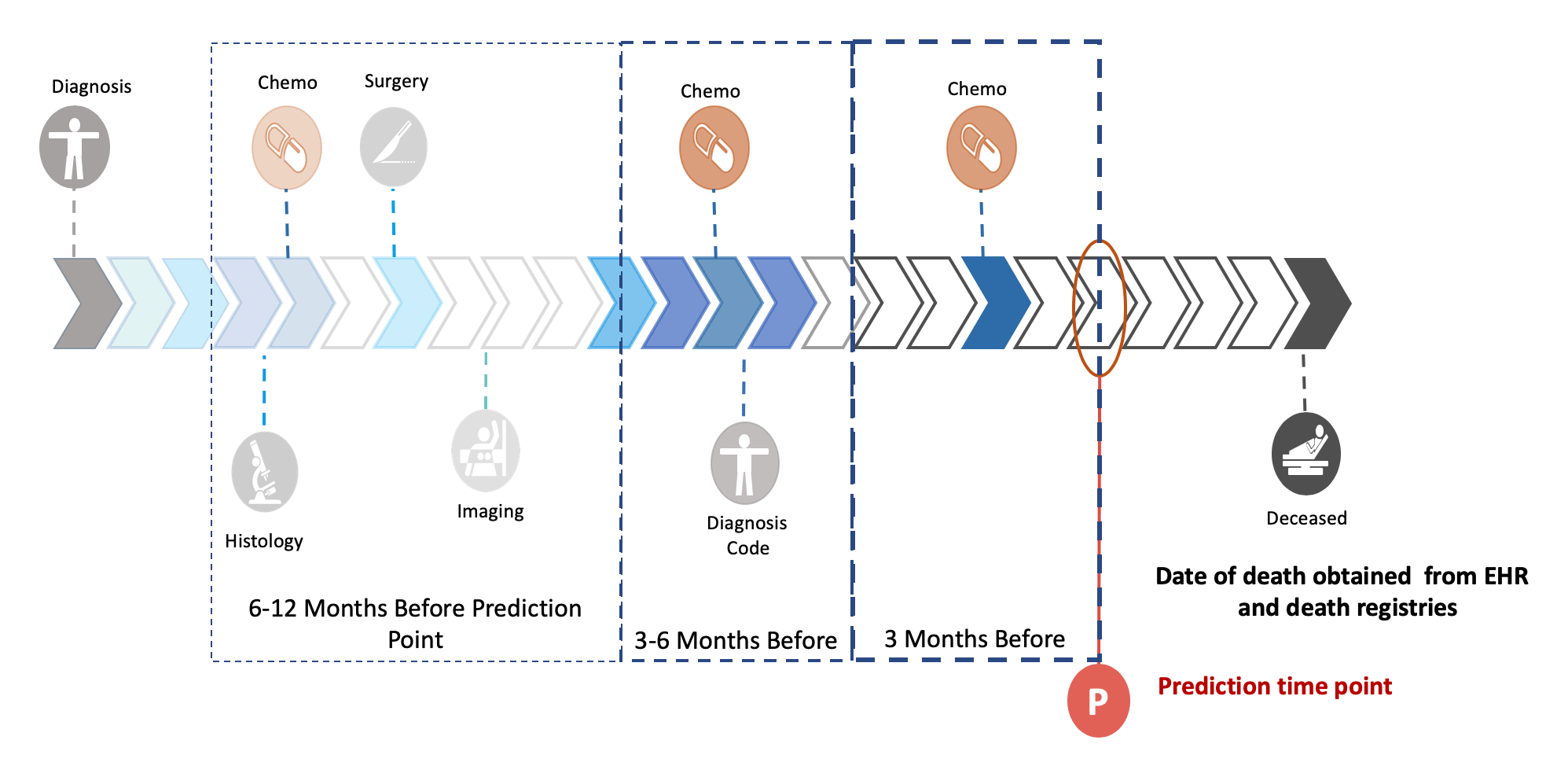
Depiction of a patient journey and features used to predict survival